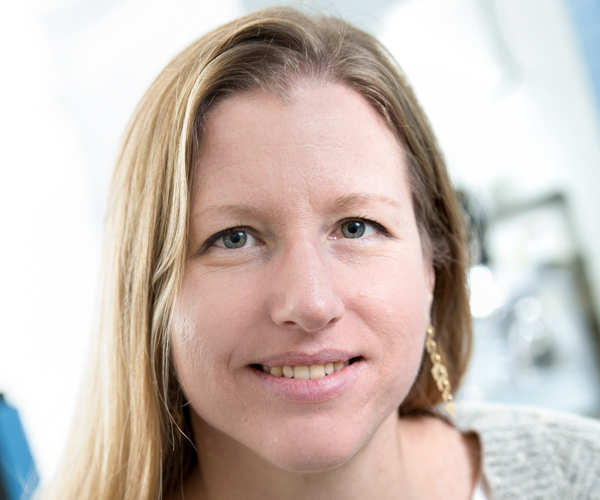
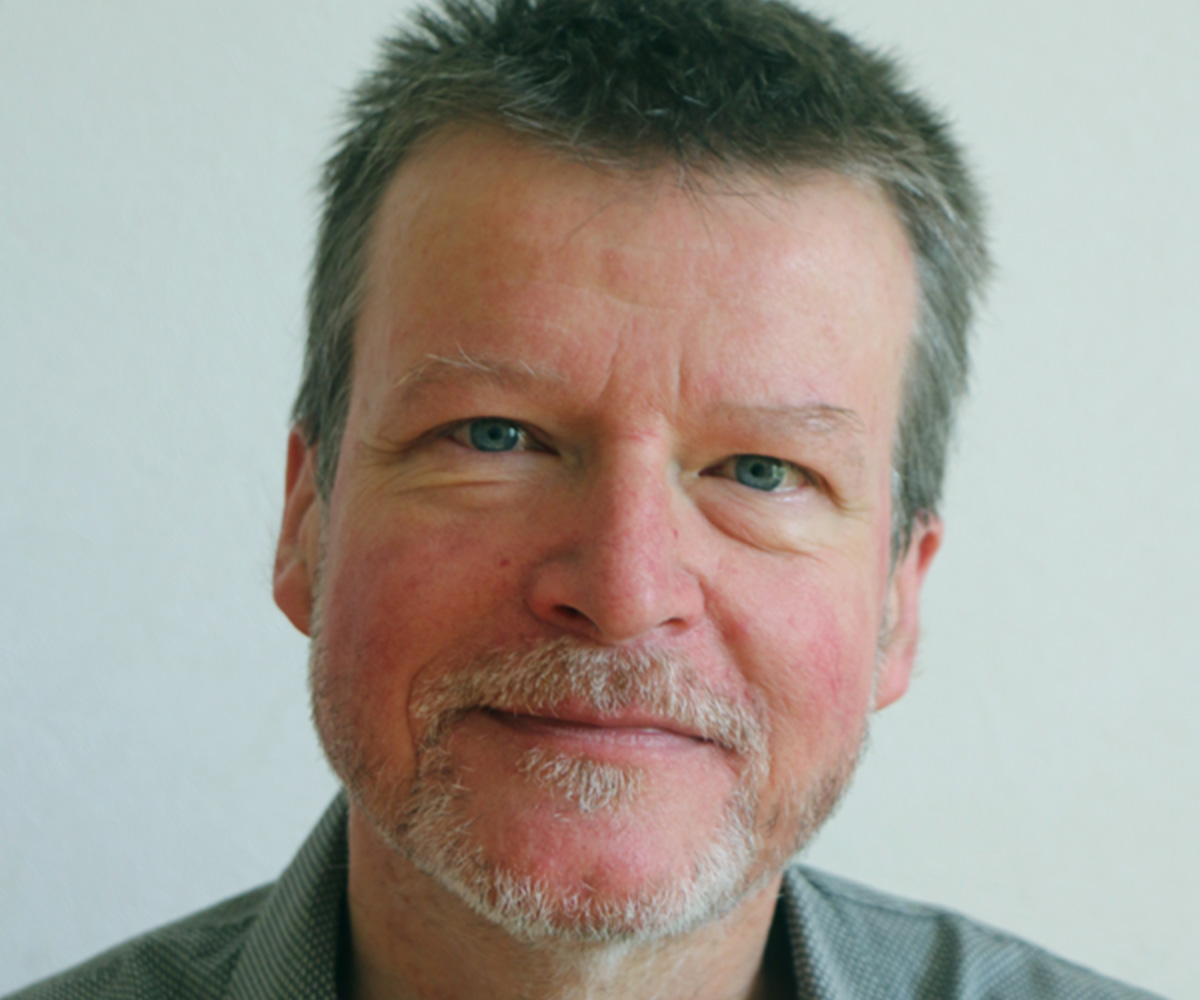
Personalized immunotherapies target neoantigens, which are generated by random mutations across the cancer genome. But prioritizing these antigens by their predicted immunogenicity—or ability to provoke effective immune responses—is a major challenge and vital to the optimal design of personalized cancer vaccines, which may only include a small subset of them. Yet methods for such prioritization that use specific criteria—like how likely they are to be presented to and recognized by T cells—have produced little consensus in their rankings or consistency in their performance across datasets. Machine learning methods, which can train algorithms while simultaneously taking into account multiple criteria, have performed much better. Researchers led by Ludwig Lausanne’s Michal Bassani-Sternberg and Markus Müller reprocessed data from three large immunogenicity screening assays from 131 cancer patients and applied machine learning to train algorithms to select the most promising neoantigens. Their approach, reported in an October issue of Immunity, accurately predicted immunogenicity across datasets and achieved a 30% improvement on the ranking of neoantigens. The algorithm also furnished useful insights on the criteria that best predict neoantigen immunogenicity. Their methods will find good use in the design of personalized immunotherapies at Ludwig Lausanne.
Machine learning methods and harmonized datasets improve immunogenic neoantigen prediction
Immunity, 2023 October 9