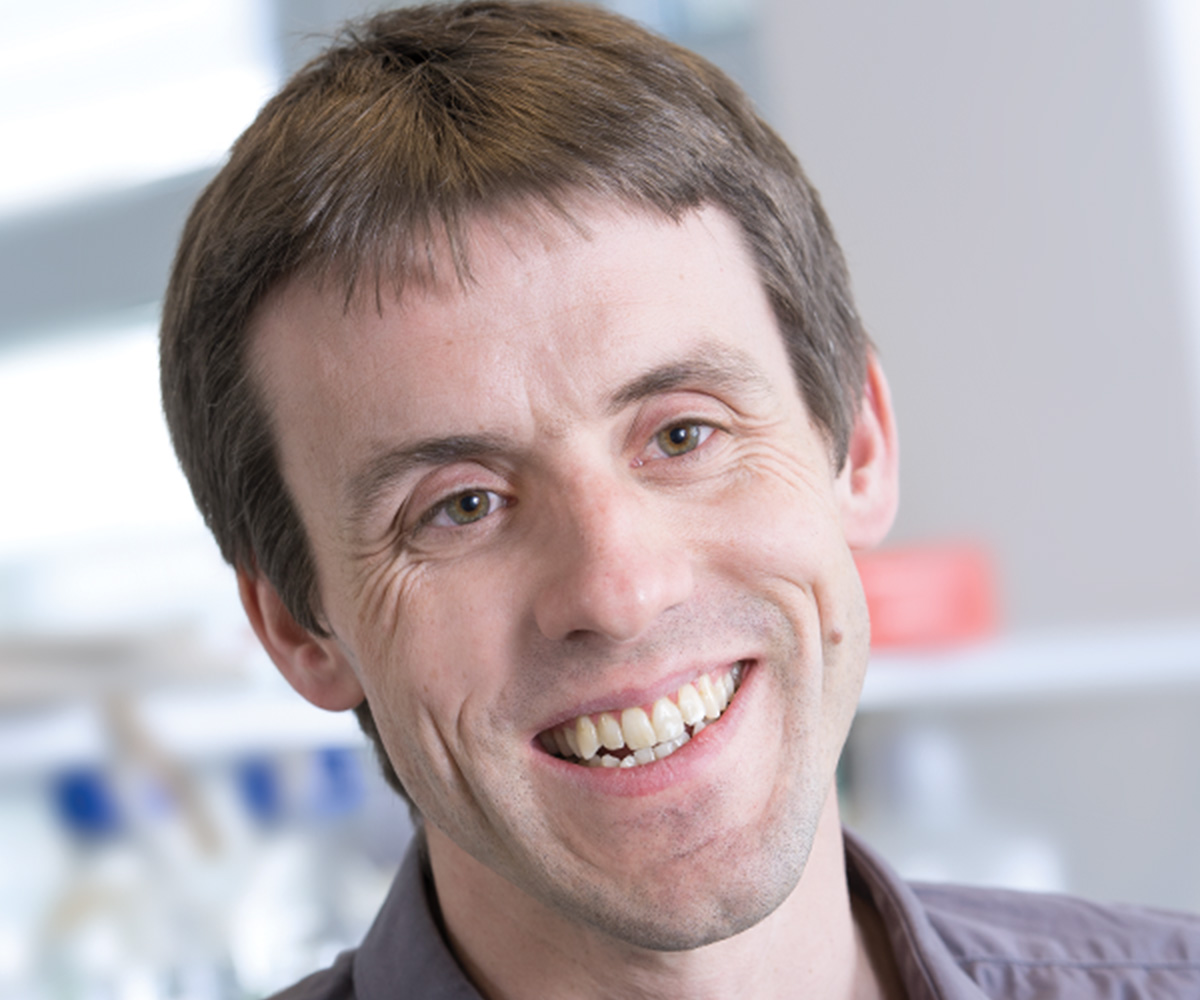
A study led by Ludwig Lausanne’s David Gfeller reported in Cell Systems in January the development and validation of machine learning algorithms (MixMHCpred and PRIME) that predict with high fidelity the antigens likely to be recognized by cytotoxic CD8+ T cells of the immune system. CD8+ T cells distinguish infected or malignant cells from normal ones by recognizing small protein fragments—or neoantigenic peptides—that are foreign or appear abnormal due to mutations that occur across the cancer genome. In humans, these neoantigens can be displayed on the surface of diseased cells by a class of proteins known as class I human leukocyte antigens (HLA-I). Yet not all suspect peptides in cells can be targeted by cytotoxic CD8+ T cells, as not all bind to HLA-I on cells, and even among those that bind to HLA-I, not all are seen by the receptors expressed on T cells. Predicting which peptides are likely to be presented by HLA-I and recognized by T cell receptors is valuable to the development of individualized cancer immunotherapies and interventions for infectious diseases. David and his colleagues applied their PRIME algorithm to identify several peptides associated with SARS-CoV-2—the virus that causes COVID-19—that are likely seen by CD8+ T cells and thus involved in immune responses to the infection. They then validated their predictions in laboratory studies. One such peptide from SARS-CoV-2 whose equivalents in other coronaviruses are also recognized by CD8+ T cells could be of value to the development of broader interventions to prevent or treat infection by these medically important viruses.
In another study, published in Immunity in April, David and his colleagues described a novel computational approach to better characterize how CD4+ T cells recognize neoantigens and predict which are most likely to elicit a functional response from the immune cells. These cells—which include the helper T cells that orchestrate adaptive immune responses—recognize antigens displayed on the surface of cells by MHC-II molecules (the equivalent of HLA-II in humans). The specificity of MHC-II molecules is not well understood, and identifying all peptides that are likely to elicit immune responses experimentally is both complex and costly. David’s team analyzed more than a half million MHC-II ligands to get a better handle on MHC-II binding specificities. This revealed new structural mechanisms of MHC-II binding to peptides and allowed the researchers to improve predictions of the antigens targeted by CD4+ T cells using their MixMHC2pred software. Their software tool, the researchers reckon, could reduce by at least a factor of 100 the number of peptides that need to be experimentally verified as potential helper T cell targets in the design of personalized immunotherapies.
Improved predictions of antigen presentation and TCR recognition with MixMHCpred2.2 and PRIME2.0 reveal potent SARS-CoV-2 CD8+ T-cell epitopes
Cell Systems, 2023 January 4
Machine learning predictions of MHC-II specificities reveal alternative binding mode of class II epitopes
Immunity, 2023 April 5